Geothermal Energy in the Era of Artificial Intelligence
At a moment when the tide of concern about climate change is reaching flood stage, it’s clear that the world is destined for a significant transformation in the production and consumption of energy. For example, in January, BlackRock CEO Larry Fink declared that “climate risk is investment risk,” and that “…there is no denying the direction we are heading. Every government, company, and shareholder must confront climate change.” Since BlackRock is the world’s largest investment firm, with more than $7 trillion USD under management, Fink’s proclamation that BlackRock will consider the environment as they assess investments may indeed be a turning point. Especially for the Energy industry.
Decarbonization efforts are gaining traction at the same time demand for electricity is increasing. Case in point: the increased commitment by automobile manufacturers to build electric vehicles. Volvo, Mercedes Benz, Porsche, Ford, and Nissan are among the companies that have announced their intentions to build more electric vehicles.
Recently, General Motors’ CEO, Mary Barra underscored the trend: "GM believes in the science of global warming. We believe in an all-electric future. It’s not a question of if, but when."
In the context of increasing reliance on electricity for buildings, transportation and manufacturing, and the search for renewable resources as a base load to complement intermittent sources and deliver the energy we need, Geothermal proponents have earned a seat at the power generation table.
Although popular perceptions might position us on opposing sides of the global climate change discussion, what can the Geothermal industry learn from our colleagues in the oil and gas industry?
Given the similarities in subsurface challenges and overlapping technologies, can their knowledge, deep pockets and vigorous efforts to transform business operations through “digitalization” yield valuable lessons for Geothermal operators?
One promising possibility is in the field of Artificial Intelligence (AI).
For the oil and gas industry, AI systems are useful at three stages of asset life.
- Early life – exploration phase
- Mid-life – planning and operations
- Mature life – data-driven expansion to obtain the most return from a reservoir or field
How can AI provide assistance to Geothermal operators?
A major pain point for Geothermal operators is cost of drilling to find reservoirs of naturally heated water, or subsurface heat sources into which water can be introduced to enhance reservoir performance. In oil and gas production, the financial risk is mitigated by the long-term value of the commodity produced. Oil is valuable, and a single successful asset can offset the cost of exploration and provide recurring value fordecades. In Geothermal, the value is inherently lower. Further complicating the problem is the fact that the drilling dynamics for Geothermal differ from oil and gas exploration. Oil and gas tend to be found in softer rock formations, whereas enhanced geothermal systems require drilling in harder igneous rock. Drilling in high temperature environments also increases costs and risks, especially in the exploratory phases. Geothermal resources such as Hydrothermal Systems, Enhanced Geothermal Systems, and Sedimentary Systems may all benefit from advanced Cognitive AI technology, especially at a time when Diverse Energy Resources (DER) are being integrated into grids by utilities and private enterprise. Predictive demand combined with flexible supply is a viable strategy for energy security, efficient production and distribution, and environmental benefits.
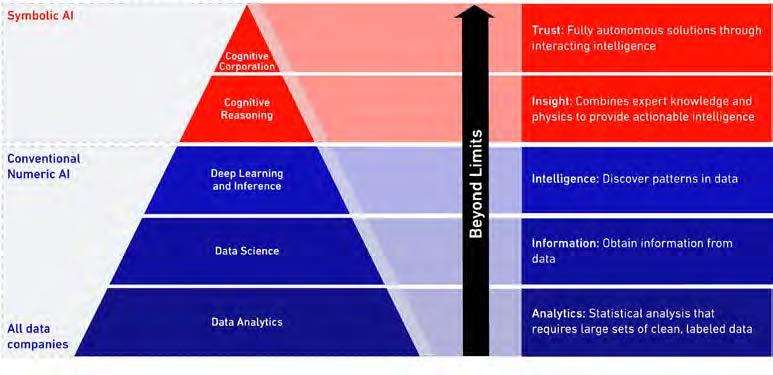
AI Technology from Space, at Work Here on Earth
Beyond Limits, a California-based AI software engineering company, is commercializing cognitive computing and AI software technologies created by Caltech’s Jet Propulsion Laboratory for NASA missions over the last 20 years. There are many similarities between the problems of deep space missions tasked to explore the unknown realms of space and those faced by energy companies exploring the subsurface.
Cognitive computing is the most sophisticated form of artificial intelligence (AI). If you think of AI as a stack of capabilities, at the base layer of the pyramid is data analytics and at the pinnacle is cognitive computing - (see figure above).
Cognitive computing emulates human reasoning and uses knowledge provided by domain experts, not just data, to understand situations, recommend actions, and solve problems. Cognitive AI systems differ from conventional artificial intelligence applications as they can adapt and become smarter over time as they interact with more experts, problems and data. Cognitive AI systems are able to operate in complex situations where uncertainty exists and data may not be as prevalent as we would like, and in such situations, they analyze and solve problems much as a human would.
Beyond purely numeric AI approaches, Cognitive AI systems incorporate symbolic reasoning in the form of knowledge bases from human experts, enabling hypothetical reasoning scenarios based on best practices to arrive at recommendations for human operators. Predictions about the most promising locations to drill are detailed in transparent, evidence-based audit trails documenting confidence, uncertainty, and risk levels. This is known as explainable AI (X- AI) to differentiate from the black-box obscurity of conventional numeric machine learning AI approaches.
The technology is maturing rapidly, and in the energy sector, Beyond Limits is developing and deploying new products for refinery optimization, managing particulates in upstream wells, assisting chemical engineers in formulating lubricants products, and advising reservoir engineers on optimized well locations and in-fill well production rate prediction. As BP (a Beyond Limits customer and investor) recently stated
“Beyond Limits codification of human expert knowledge and use alongside machine learning has the potential to help the energy sector improve operational insight, improve safety, further optimize performance, and introduce additional process automation.”
Ultimately, cognitive systems will function in coordination across business domains, fostering collaboration between experts in subsurface and drilling to improve accuracy and efficiency.
To help manage the operations of LNG power plants, especially in grids that need to balance supply and demand with a mix of intermittent power sources like solar and wind, Beyond Limits is developing a system for the worlds’ first power plant guided by Cognitive AI technology. The system will be installed in a new LNG plant to be constructed in West Africa to drive industrial development.
Three AI Use Cases from Oil & Gas with Potential for Geothermal Energy
Subsurface Modeling and Drilling Location Targeting
Solution
These solutions combine machine learning data into the cognitive reasoner with reservoir engineering knowledge bases.
Result
The Beyond Limits system identifies high- productivity wells in physically meaningful reservoir areas in a matter of minutes to hours, rather than traditional approaches that can take days to weeks. The solution rapidly integrates new data and provides fresh recommendations, dramatically reducing cycle time while increasing the number of plausible development scenarios that may be investigated.
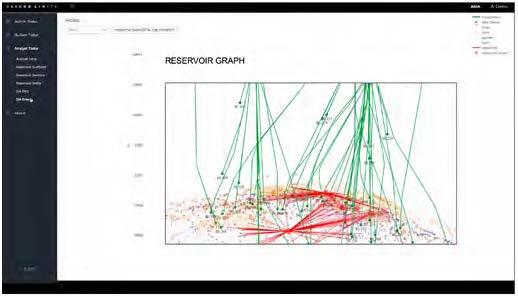
Well Health and Productivity
Problem
In oil and gas wells, high production rates can mobilize particulates into the production stream, damaging equipment over time, decreasing production, and increasing maintenance costs. Operators desired an AI monitoring solution that uses engineering knowledge and heuristics to predict or detect potential particle problems in advance, then recommend changes in operating conditions at the well-level and field-level to meet contractual production constraints and minimize particle production to ensure that volume targets are met.
Solution
Beyond Limits employs state-of-the-art machine learning and data science frameworks to evaluate risk associated with well performance such as particle production. Engineering knowledge bases are leveraged by our cognitive reasoner to determine production risks (as well as mechanism and volume levels) to suggest changes to improve operating conditions and efficiency.
Result
The Beyond Limits well health system detects major particle production problems with near 100% accuracy, which can significantly reduce downtime and operating costs. The technology also evaluates potential risks in future plans, enabling operators to leverage in-field experience for optimal well operation and production planning.
Refinery Production Management
Problem
Every day, operations teams strive to manage systems, processes and team performance to optimize outcomes. Unfortunately, the operational state of a refinery often deviates from the assumptions used in the planning process and it requires human intervention and timely decisions to remediate throughput, quality, reliability and profitability risks and return the process to desired performance ranges.
Solution
Because refineries operate around the clock, Beyond Limits developed an always-on refinery operations advisory system. The cognitive decision support system empowers operations teams to improve operations plans through better transparency, communication and collaboration. The system stores, classifies, and leverages lessons learned so operators can uniformly apply best practices at the planning, operations, and management levels of the organization.
Result
Cognitive AI automatically identifies off- plan behavior. And, as data is collected in the system over time, it learns to better predict this behavior. Finally, the system provides guidance and remediation recommendations at the point of decision, based on optimization strategies and actions provided in the plan
Early and Inevitable
We are in the early days of applying artificial intelligence to help manage and improve performance in oil and gas exploration and production. While there are parallels between oil and gas operations and Geothermal operations, AI systems have not yet been customized to meet the unique demands of the Geothermal industry. Given the strong potential evident in today’s new AI systems for energy production, it’s inevitable that machine learning and AI will become integral components of the Geothermal industry.
Introduction to Cognitive Artificial Intelligence – Beyond Conventional AI
From a high level, artificial intelligence can be broken into two broad camps: Numeric AI (training from data), and Symbolic AI (education from knowledge). With conventional Numeric approaches, you train the system by providing example data (e.g., time series, images) of what you want it to classify. So, if you want it to recognize a cat, you supply it with thousands of different pictures of cats. With Symbolic approaches, you educate it by providing knowledge to understand the subject. So, if you want to create an AI doctor, you supply volumes of medical textbook knowledge that the system ingests.
For data-centric problems, numeric AI has accomplished great things in a variety of areas. However, conventional numeric AI machine learning and neural nets alone are insufficient to solve many complex problems where expert knowledge is a required component of reasoning and decision making, and when results need to be explainable, data is limited, unreliable, or misleading. On the other hand, symbolic reasoning alone is insufficient when data sets are large and clues from the data are needed to solve the problem.
Disadvantages of Using Only Numeric AI
- Brittle Solutions: Numeric approaches break when the same question is asked in a slightly different manner or the data changes just a little.
- Requires Pristine Data: When data is less than ideal, these systems do not fail gracefully but completely break down because they are unable to reason.
- Cannot Explain Answers: Artificial neural networks are statistically based, therefore their decision processes are not easily invertible. In other words, they are black boxes that cannot explain how they arrive at an answer.
For complex problems, AGI (Artificial General Intelligence) is the desired approach, but it is a long way off. Nevertheless, at Beyond Limits, we are working on getting there sooner than you might think.
Meanwhile, how can we solve complex problems today?
The newest generation of AI applications address high-value assets that require human-like understanding of complex domains with systems that can adapt to uncertainty in both their knowledge and data, supporting their answers with human-understandable audit trials (also known as Explainable AI or X-AI).
This requires the integration of different learning and adaptation techniques to overcome the limitations of the individual technologies and achieve synergetic effects through hybridization of symbolic and numeric technologies. At Beyond Limits, we have seen the need for a hybrid approach combining numeric machine learning technologies and symbolic reasoners for cognitive intelligence – the stepping-stone to AGI.
The machine learning technologies we use include artificial neural networks, deep learning, Supportive Vector Machines (SVMs), Bayesian networks, and genetic algorithms. Symbolic technologies include knowledge graphs, case- based reasoning and deductive, inductive, abductive, defeasible, and modal reasoning.
As we further develop our hybrid cognitive AI approaches combining the best of both worlds, the result will be self-organizing systems of AI- working-with-AI-working-with-Humans, which orchestrate intricate collaborations between specialized intelligent agents to solve highly complex problems requiring diverse shared expertise.